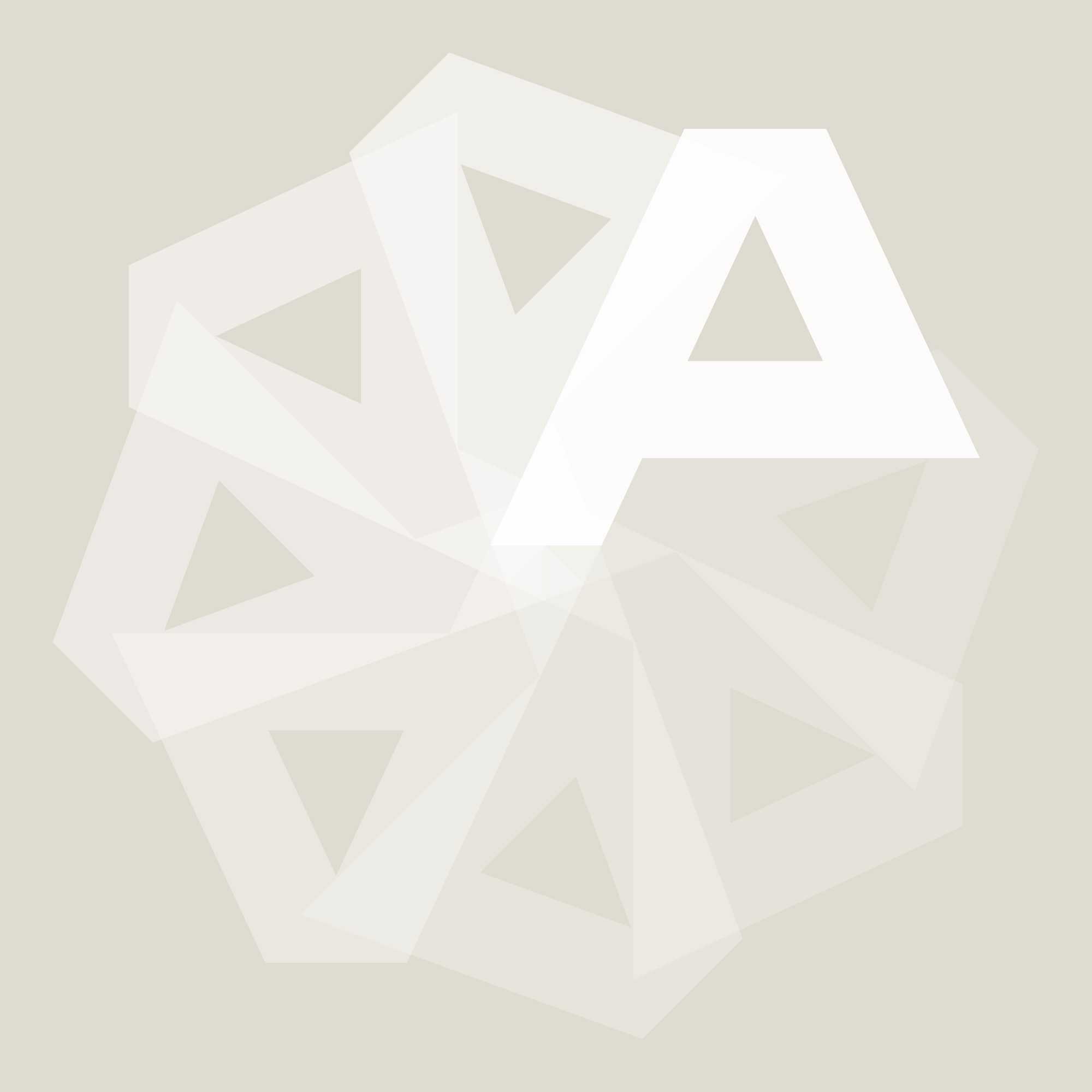
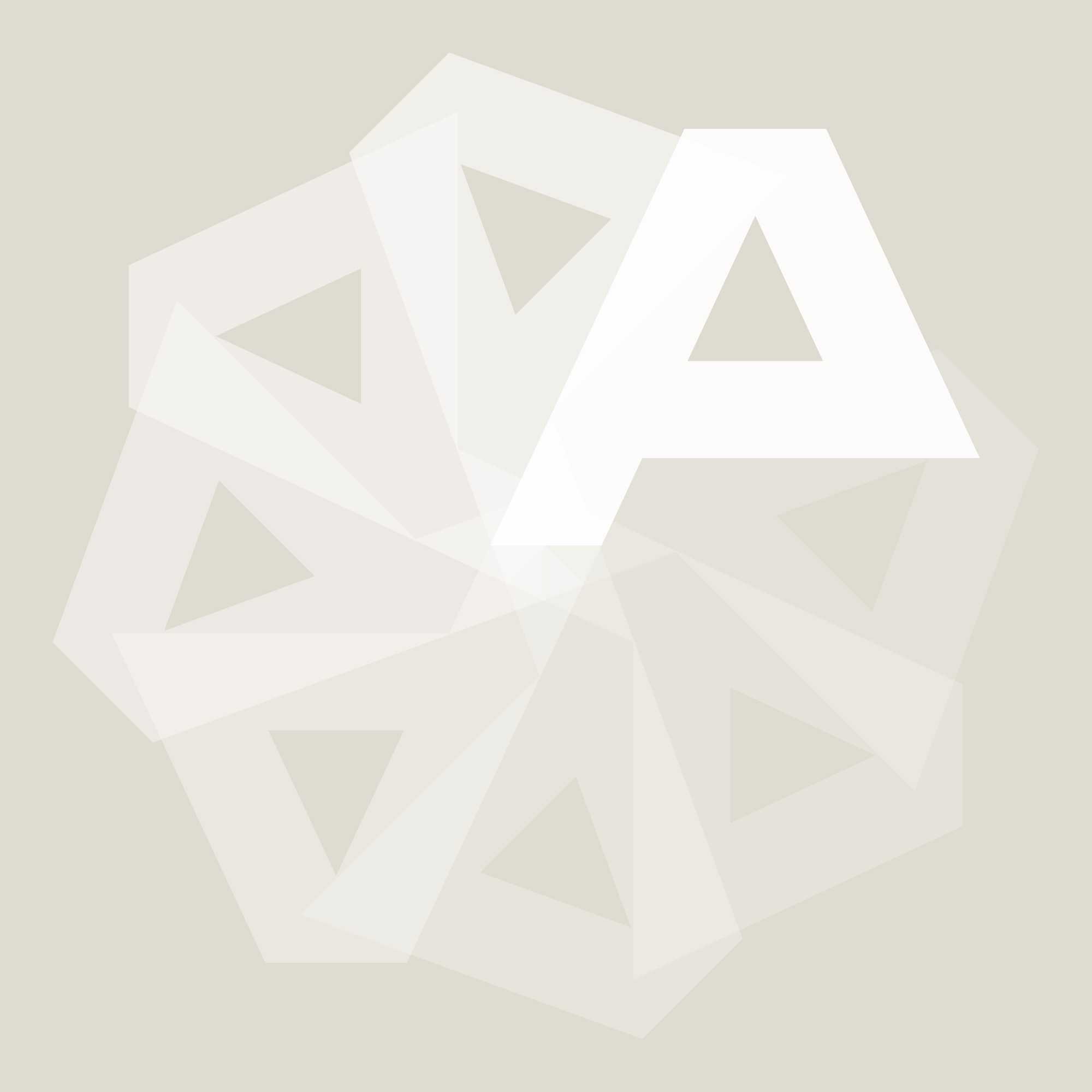
Predictive algorithms are mathematical models used to predict future events, outcomes, or patterns. They are used in many different fields, including medicine, finance, marketing, and even in your mobile phone or voice-activated device.
Predictive models use language as text, images from sensors, or transactional quantitative data from the past. The source of data for predictive models can be any data that can be used to predict future events. This includes data from past, current, or future events.
But how many predictive models are there? There is no absolute answer as the number of predictive models available depends on the specific application or industry. However, some commonly used predictive models include linear regression, logistic regression, decision trees, and support vector machines.
Many industry verticals employ predictive models as powerful tools that help us make better decisions and plan for the future. Their analytical power helps us understand how likely something is to happen. For example, can a CEO make better decisions about what to do next? Can we optimize a supply chain to reduce waste or dead inventory? Can a business increase production due to new demand surges and consumption patterns? Or can a business predict real estate value.?
Predictive models can improve optimal outcomes, but are prone to disruptions, mainly for businesses that rely on global and diversified sources. These deviations can lead to strategic surprises if business leaders are not prepared.
Predictive models can be perturbed by geopolitics in several ways. Like the effects of COVID-19, and cascading issues like logic disruptions. For example, if a model is based on historical data of a target variable, then a change in geopolitical conditions could invalidate the model. Alternatively, if a model is based on current data, then a change in geopolitical conditions could cause the model to make inaccurate predictions. We live unstable lives in unstable conditions. Why can’t your models adapt to change? The sources and reasons could be many and varied, paralyzing your data science and management teams.
There are many ways to improve predictive model robustness and statistical procedures; some standard techniques include feature selection, feature engineering, hyperparameter tuning, model selection and ensembling.
Data, information, knowledge, and wisdom
Data is the law and the essence of any predictive model; thus, data science teams should understand that the newcomer’s mistake is to fail to plan enough time to acquire and clean the data. It is a best practice to spend close to 60% to 80% of a project’s allocated time shaping the data. You should be asking what-if questions, and consulting with domain-specific consultants — tap into the wisdom inside your organization, the cool data science team will only know so much.
Through the data inspection process, some ways to improve the robustness of data sources include taking extra steps to ensure data is of high quality, using a larger dataset, using a more diverse dataset, and using a more balanced dataset.
The work of data science is never done, and model iterations is what should keep a data science team busy. Geopolitical signals are examples of critical events that can have real-time influence of streaming data sources. The distress of these critical events might have short term and long-term effects. Teams should be looking for the early onset of model drifts throughout a model’s lifecycle and deployment.
Organizations should never expect to “bake a predictive model and forget about it” after deployment. Instead, they should expect to monitor performance regularly and retrain their model as new data comes in.
There are countless ways to improve predictive model robustness, and too many considerations, but here are some examples:
- If you use natural language data such as text from news articles, finetuning natural language processing (NLP) and sentiment analysis might improve accuracy, consider how fake news and misinformation impact predictions. Remember these contain information about the past, present, and future. Filter for quality of signal versus noise.
- Monitor your first-party data closely, it allows teams to adjust models to better learn the new patterns in your business that may signal new behaviors.
- Simulations and simulacra are often not in data science playbooks, but what if there was a severe drought and the crops failed thousands of miles away? What if a hurricane disabled all the ports in a strategic location? How does a war disrupt your supply chain? Or how do you integrate critical event data into your model?
- Making important variables more robust by training your predictive model to new and unseen data from critical events is necessary for any resilient organization.
- Embrace innovation of a more robust model, such as a deep neural network, which might better learn complex patterns in data and be less susceptible to overfitting.
- Dimensionality reduction— Reducing the number of random variables under consideration can be achieved either by selecting a subset of the original variables or by projecting the original data set onto a lower-dimensional space.
In data science, there is no right or wrong, there is only data. Data science is the outcome of experiments and perseverance, but eventually, your team learns how to adapt to the world’s current affairs and continuously reevaluate models’ risks and identify difficult predictions that are valuable.
I encourage you to remain engaged with data science teams and monitor your predictive model’s performances through machine learning operations and become an executive in the loop and improve and increase your predictive model’s trustworthiness.
And remember the age-old adage, past performance does not necessarily predict future results, but we can improve the odds.