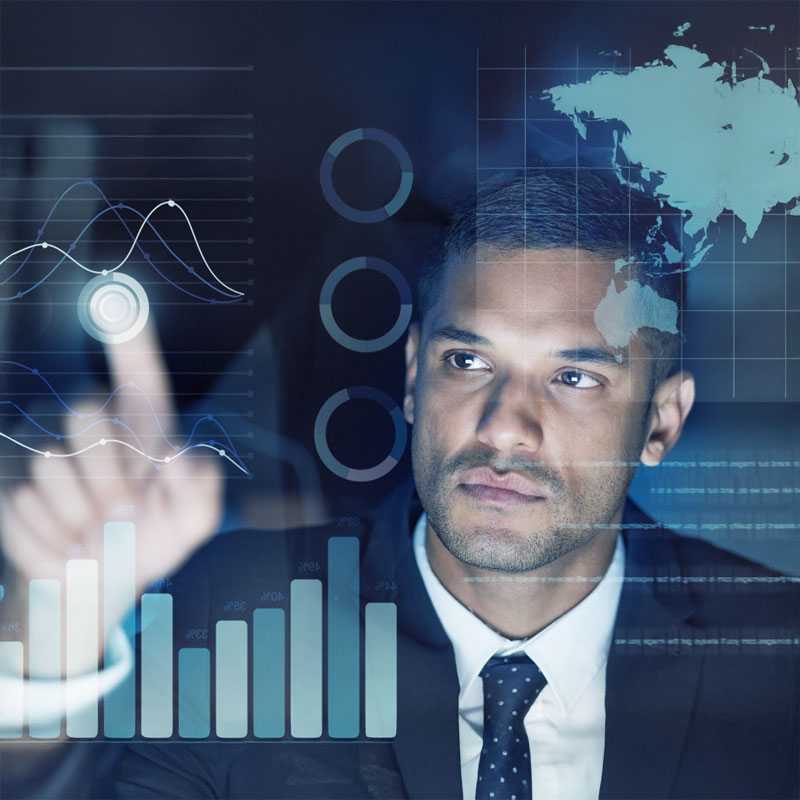
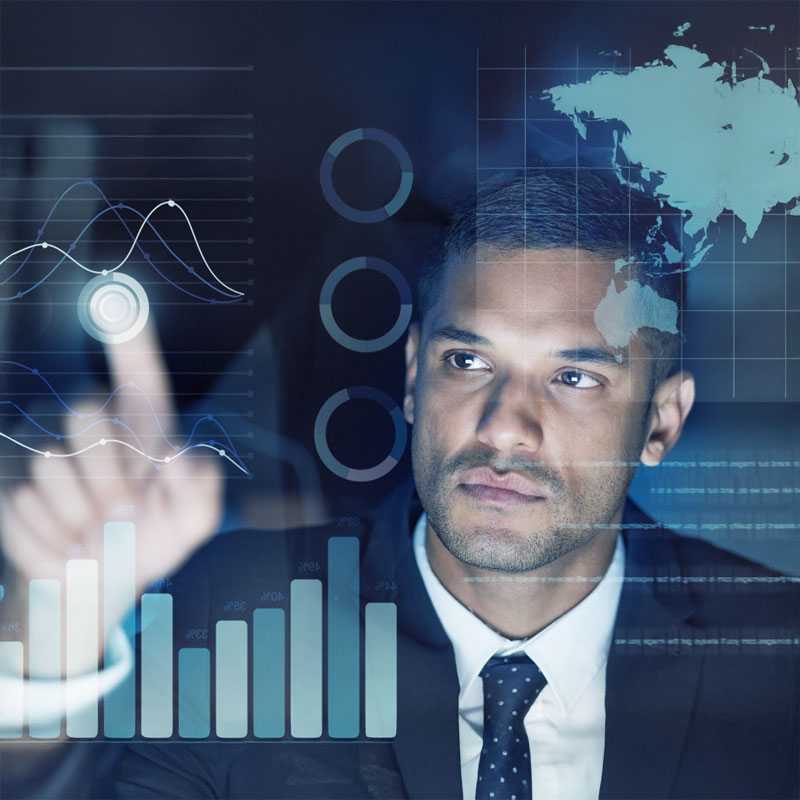
In today’s rapidly evolving business landscape, traditional market research methods such as in-person focus groups, extensive surveys, manual data analysis and analyst reports can be slow, labor-intensive and costly. Recent breakthroughs in generative artificial intelligence (AI), including large language models (LLMs), multimodal models and transformer architectures like ChatGPT, Claude or Gemini, present an opportunity to enhance market research capabilities by delivering faster and more extensive insights than ever before.
However, multimodal models also bring significant challenges, drawbacks and ethical considerations to the forefront that require careful navigation. To maximize the potential of AI while addressing challenges, market research teams must adopt a balanced and careful approach.
The Challenges of Using AI in Market Research
Integrating AI into market research presents several challenges, that can risk the reliability and integrity of AI-generated outputs.
- Algorithmic bias. AI systems can generate biased outcomes that mirror and perpetuate societal biases, like historical and social inequalities. Bias can originate from the initial training data, the algorithm itself or the predictions it generates. In the context of LLMs, which is the main type of AI technology that is being implemented, data or the text used for training is uneven. This means the training data is not uniformly distributed across various subject areas, languages or conceptual frameworks, leading to potential biases in the model’s knowledge and outputs.
- AI bot infiltration. AI bots pretending to be real survey respondents can impact data integrity and wreak havoc on online research. These bots often powered by the latest large language models, often are indistinguishable from humans posing significant challenges to maintaining the authenticity and reliability of survey data.
- Excessive automation and dependency. While AI offers powerful data processing capabilities, over-automating without human oversight can compromise the validity of insights. AI can process large-scale data but lacks the emotional intelligence, contextual understanding and judgment of experienced analysts. In scenarios involving open-ended responses or interviews, AI may misinterpret nuances in human behavior and cultural subtleties, potentially compromising the validity of insights without human supervision.
- AI confabulation. Confabulation refers to the generation of plausible sounding but potentially inaccurate or fabricated information, a common characteristic seen in LLMs when they produce responses based on limited or incomplete knowledge. Confabulations are very characteristic of human memory. Similar to LLMs, our brains store knowledge through weighted connections, using them to reconstruct events. Recent events are generally accurately reconstructed, whereas older events often result in inaccurate details unless rehearsed frequently. Interestingly, confidence in these incorrect details remains remarkably high.
- Data privacy concerns. Handling sensitive data with LLMs poses data privacy challenges. Market researchers must navigate the complexities of handling large volumes of personally identifiable information (PII) while ensuring compliance with stringent privacy regulations such as General Data Protection Regulation, California Consumer Privacy Act and other relevant laws. The use of LLMs to analyze market research data such as survey data, open-ended responses or interviews can raise data privacy concerns such as passive privacy leakage by unintentionally exposing sensitive data to LLMs by entering such information into the chat interface.
Guidelines for Maximizing Value
To maximize the benefits of AI in market research while addressing these challenges, market research teams can consider the following guidelines:
- Mitigating algorithm bias. Confirm AI-generated results through cross-validation with traditional methods and multiple AI models. Include contextual analysis that takes into account cultural and social nuances affecting AI interpretation. Human reviewers should verify AI-generated insights, using AI to support rather than replace human decision-making.
- Tackling AI bot infiltration. Implement rigorous measures such as advanced bot detection techniques, regular audits and respondent authentication methods to maintain data integrity. It’s crucial to recognize that this is an ongoing effort, especially as generative AI becomes increasingly capable and its responses become barely distinguishable from those of humans.
- Preventing excessive automation and dependency. Integrate human expertise throughout the AI process to verify outputs, interpret findings and provide additional context where AI may be inadequate. Human oversight ensures accurate interpretation and effective application of AI-generated insights.
- Addressing AI confabulations. Crafting clear and detailed prompts is essential for minimizing AI confabulations. By providing precise instructions that define the context, specify desired details and cite sources, prompts can effectively guide AI to generate accurate responses, minimizing room for misinterpretation. This approach enables AI to focus on producing relevant and reliable outputs by avoiding assumptions or fabrications.
Despite advancements in AI, integrating human review remains critical in detecting AI confabulations. Human fact checkers can identify and correct inaccuracies that AI may overlook, thereby ensuring the reliability of outputs. It’s important to note that LLMs vary in training and capabilities, so conducting due diligence when selecting the most suitable model and understanding its knowledge gaps can enhance precision in outputs.
- Handling data privacy concerns. Practice data sanitization techniques before inputting data into LLMs to remove sensitive data. Limit the context provided to the LLMs during inference. For example, you can truncate or mask parts of the input text to avoid revealing sensitive details. It is important to note that LLMs have differential privacy.
The Bottom Line
As we venture into the AI-enhanced landscape of market research, we’re faced with a potent mix of opportunity and challenge. This digital dynamo promises to supercharge our insights, but it comes with a user manual we’re still writing. By blending AI’s analytical prowess with human expertise and ethical considerations, we can harness its potential while sidestepping its pitfalls. In this new era, success lies in striking the right balance—leveraging AI as a powerful ally while keeping our human touch firmly on the wheel of market understanding.