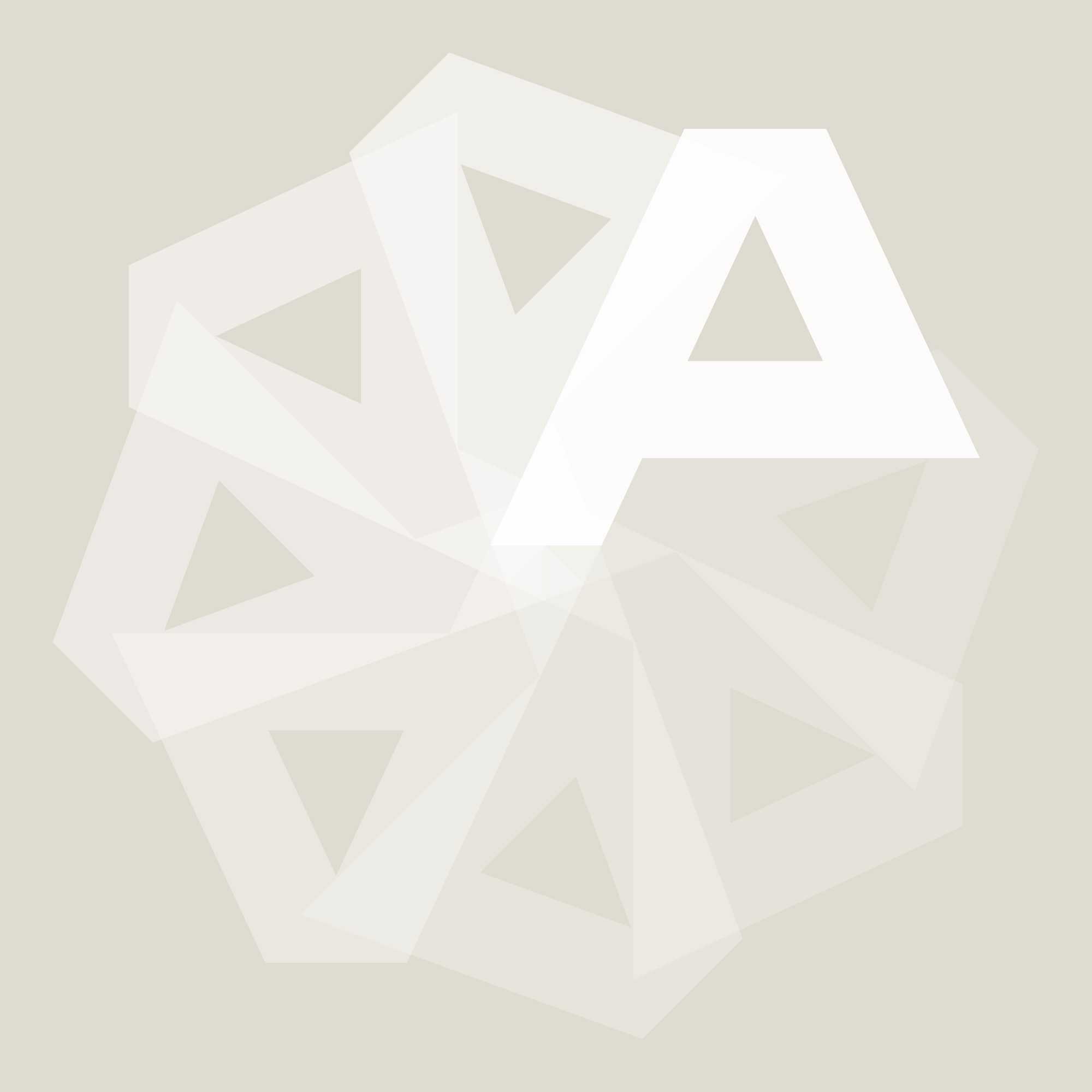
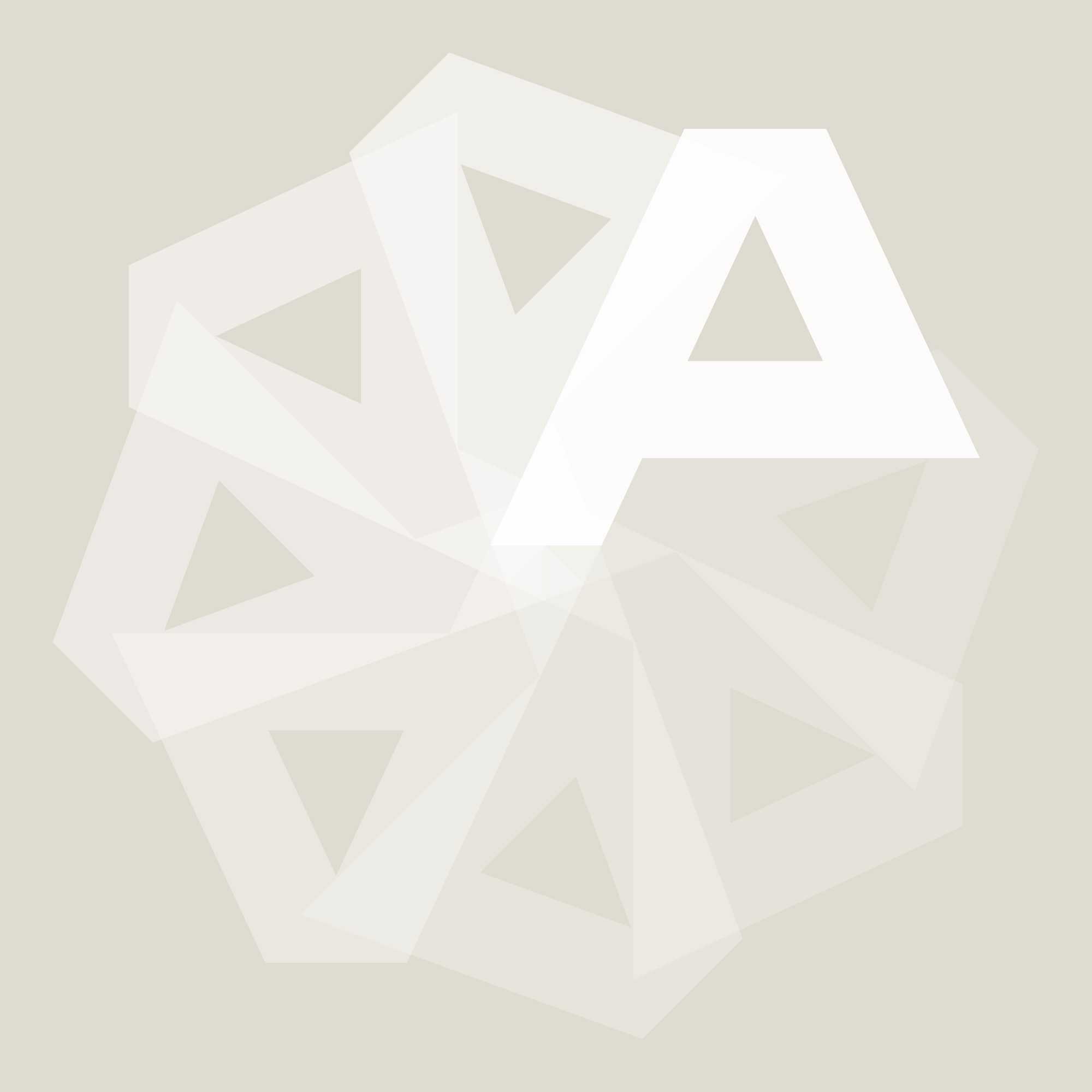
When can doctors marshal the collective wisdom of all clinicians to inform the care of every patient?
What if every medical decision is guided by the collective wisdom of all clinicians and the outcomes data gleaned from diagnosis and care of billions of patients around the world?
This may sound like a pipe-dream. Nevertheless, this is the tantalizing promise of machine learning in medicine powered by digital technology and Artificial Intelligence (AI), as discussed in an excellent review article in the new issue of The New England Journal of Medicine.
However, despite the potential promise of machine learning, the adoption of machine learning in medical practice is surprisingly slow, according to the authors from the Department of Biomedical Informatics, Harvard Medical School.
There are a number of challenges that will need to be overcome to accelerate the broader application of machine learning in medicine:
Linking siloed data sets: Building machine-learning systems requires training with data that delivers an integrated, longitudinal view of the patient. As the authors point out, many data sets are currently housed in siloed systems such as EHR, medical imaging archives, clinical databases and health registries, payer claims databases, patient data in apps and etc. Aggregating and linking all the diverse data sets in a unified platform is a highly complex process.
Ensuring the quality of data: Making the right decisions about patient care-based AI and machine learning requires that the data has quality. The CEO of Novartis, Vas Narasimhan, recently explained the painful process of applying AI and machine learning (ML) in pharmaceutical R&D:
“The first thing we’ve learned is the importance of having outstanding data to actually base your ML on. In our own shop, we’ve been working on a few big projects, and we’ve had to spend most of the time just cleaning the data sets before you can even run the algorithm. That’s taken us years just to clean the data sets. I think people underestimate how little clean data there is out there, and how hard it is to clean and link the data.”
Addressing clinical variations: Doctors often say that every patient is unique. Yet, aggregated sets of data across many patients typically show that the majority of patients fall inside a normal range with few outliers. Yet, applying machine learning has shown to be a challenge due to regional variations in medical practice, cultural bias and the constant speed of learning and insights from newly published evidence.
Changing physician behavior: Modifying clinician behavior may be one of the biggest challenges. So far, the physician experience with the large-scale introduction of digital technology in everyday medical practice has not been very positive. The implementation of electronic medical records led to disruption of clinical workflows and a frustrating experience with increased requirements for data documentation. Generating value from large-scale ML in medical practice will require that every clinician and their staff routinely generate and provide high quality information about patient diagnosis, treatment plans and outcomes – in other words, more data collection and processing. This will require that physicians are motivated, understand the clinical value and utility of digital and get reimbursed appropriately for their efforts.
Communications to build confidence: AI and ML in medicine can easily fuel perceptions of “Big Brother Medicine,” concerns about patient privacy breach and limitations of physician freedom and patient choice. It will be important to articulate the benefits of these novel technologies on patient care and demonstrate the value on improved clinical outcomes from actual implementations of ML in medical practice.
There are reasons to be optimistic and excited about the promise of machine learning in medicine, but the path and speed towards wider implementation is hard to predict. It will depend on many factors, such as the ability of the medical community and the health care industry at large to proactively lead the transformation towards adoption.