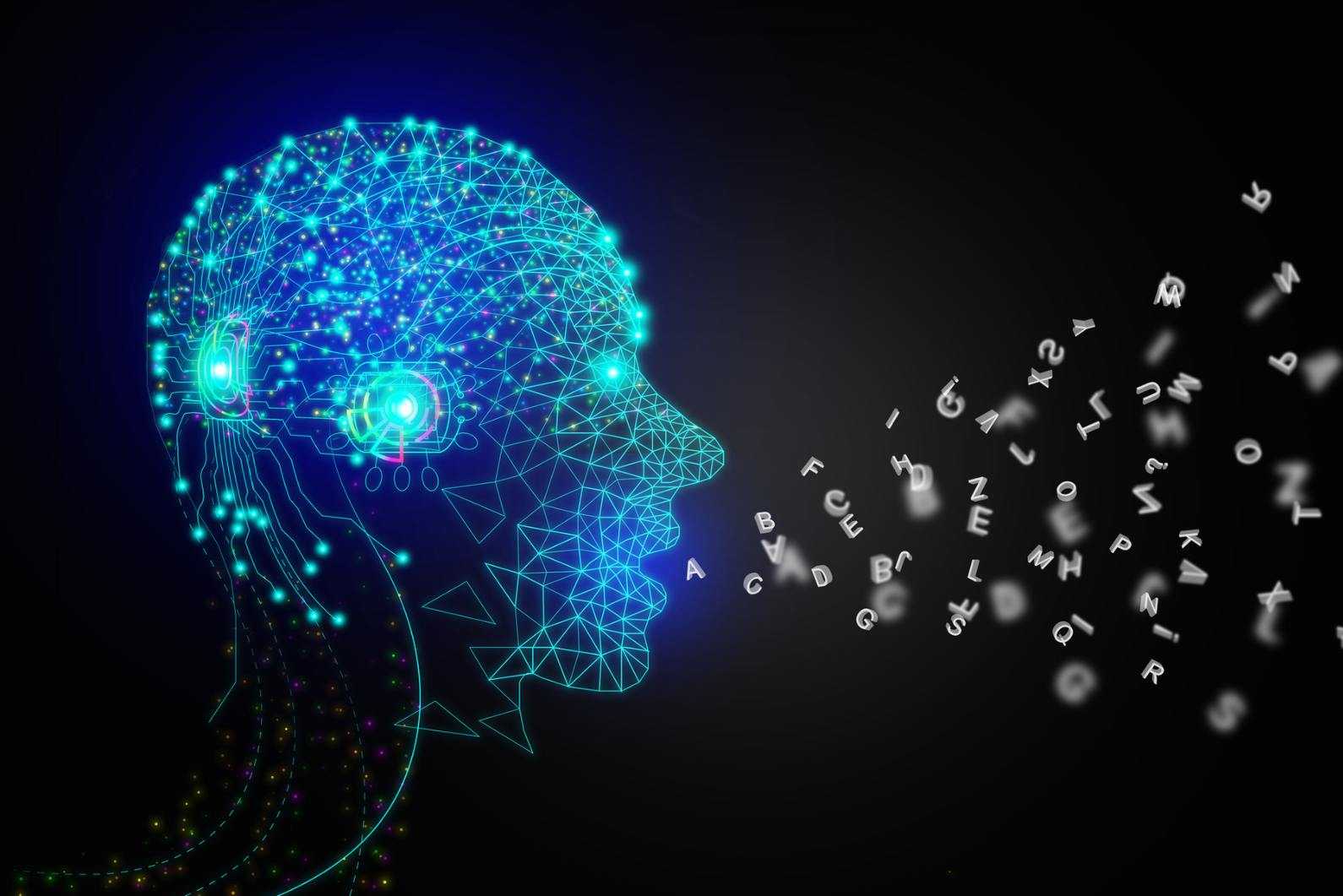
Multilingual, Not Monolithic: Why Diverse Language Data Matters for Generative AI
June 3, 2024
APCO Intern, Simone Nikitina, authored this piece.
Much attention is being paid to generative artificial intelligence (AI) as its prevalence and capabilities grow. Accordingly, generative AI is increasingly being integrated into office workflows: nearly two-thirds of office workers currently use generative AI at work or plan to. While many companies opt for commercial models like Anthropic’s Claude or OpenAI’s ChatGPT, more companies are increasingly developing proprietary generative AI models to meet their specific business needs. To harness the full potential benefits of these AI tools, companies must prioritize training their models on data from a range of languages.
Many of the most cutting-edge capabilities of generative AI, such as speeding up life-saving medication development, are powered by transformers. Transformers are neural networks that discern patterns in huge amounts of data, from which they learn contextual information that they apply to future tasks. Despite advancements in transformer-based models, a significant performance gap exists between “high-resource” and “low-resource” languages.
Out of the more than 7,000 world languages, high-resource languages are those with abundant and quality text data. Because of the large amount of data available to learn from, generative AI models perform exceptionally well in high-resource languages. English is the highest-resource language because of the copious amount of English language text on the Internet, where it is readily available to engineers. Low-resource languages, on the other hand, have small quantities of quality data on the Internet—leading to poor performance by generative AI in these languages.
Since the Internet contains copious English language data, sophisticated generative AI models can be developed by using English exclusively. These models may struggle to perform accurately and reliably when receiving prompts and producing outputs in low-resource languages. By contrast, diverse language training data helps AI models generalize better across languages, leading to more accurate and reliable performance, especially in multilingual environments. Multilingual models have been shown to adeptly synthesize data from high- and low-resource languages for overall performance that is greater than the sum of its parts.
There are many benefits of using AI tools with strong and diverse multilingual capabilities in corporate settings:
While sourcing and curating training datasets that include lower-resource languages require effort and intentionality, the long-term benefits of using multilingual AI tools in the workplace are profound. Companies should use linguistically diverse training data when developing their own AI tools to realize generative AI’s full potential to impact internal and external operations. Within organizations, linguistically diverse generative AI tools can improve communication, promote inclusivity and belonging among employees and enable knowledge-sharing within organizations. By embracing multilingual AI, businesses can pave the way for a more inclusive, efficient and globally connected workforce.