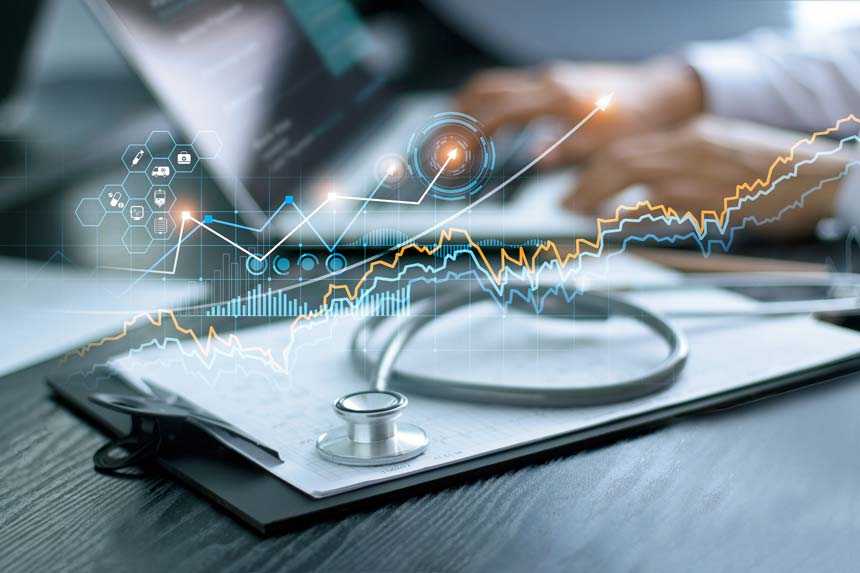
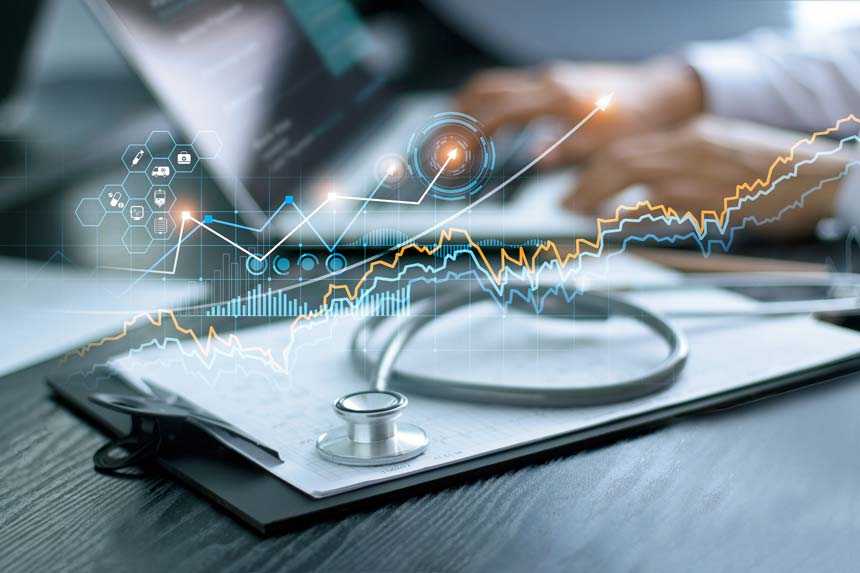
For over 25 years, I’ve been considered an expert in my field of surgery. I used to think I knew the best treatment for each patient. But as I learned about, and then applied, the principles of data science to real patient care, I’ve realized how much better our decision-making process in healthcare could be if we had a data and analytics infrastructure that could generate not just predictive algorithms but predictive algorithms for good. However, algorithms generated from big data can reinforce discrimination, racism and inequities all too common in our world.
Some algorithms are designed for self-serving purposes, clearly not intended to help all people. Examples include attempting to manipulate voters, maximize the price of an airline seat, maximize the profit from a stock trade and win baseball games. These examples come from companies and organizations that use algorithms in competition to win or make more money. But these algorithms do not result in doing good to improve our world. As soon as other competitors develop their algorithms, a competitive advantage is lost. Although they came close using algorithms described in the book and movie Moneyball, the Oakland Athletics never won the World Series using algorithms. As other teams learned to use algorithms and had larger budgets, the smaller market teams, like the Athletics, were less competitive again.
On the other hand, there are many algorithms developed to do good but lack an understanding of data science principles that could benefit all people. No algorithm is perfect, but there are ways to design algorithms that can improve outcomes for all people over time.
Decentralize the Data
Using a fundamental principle of data science to create algorithms for good in healthcare, we need to start by decentralizing these efforts in each local environment in the context of any whole, definable patient care process. Decentralization is a critical first step because centralized algorithms may contribute to unintended harm by poorly representing populations at the margins of society. Centralized datasets produce averages but lack insights.
Network Learnings
Decentralizing our data in healthcare leads to the ultimate potential—networked or ensemble learning. Once the learnings and algorithms are in the context of each local environment and a whole, definable patient care process, they can be combined with other learnings and algorithms. By combining many algorithms generated from the same process in different local environments, the predictive ability of the algorithms can be optimized.
Identify What Matters
Over time, as learnings are shared with each local clinical team, the goal should be to identify “what matters” the most to outcomes that measure the value of care. I first learned how to develop algorithms for good by applying these principles to real patient care with a small, diverse hernia team through trial and error. As we were learning to apply these data science skills, we found that we were not measuring one of the most important factors predicting outcomes for a surgical procedure: a patient’s neuro-cognitive/emotional state prior to surgery. When a person suffers traumatic experiences (violence, sexual abuse, financial stress, psychological abuse, multiple surgeries, etc.), there can be a neurophysiologic change in the brain that leads to a chronic stress state. This chronic stress can negatively impact the hormonal and immune systems and can increase inflammation in the body. When we measured the impact of that factor, it was much more important than many other factors we were measuring. It was humbling to realize there were other factors more important than my surgical technique in predicting outcomes.
Implement Improvement via Human-Computing Symbiosis
Another critical step requires algorithms to be interactive and curated by small, diverse teams in each local environment. This human-computing symbiosis is how algorithms for good can achieve sustainability in improving any complex healthcare problem and other challenges in our world. Passive algorithms, used to improve performance in rules-based applications, are not sufficient for complex problems. Addressing complex problems will require interactive algorithms applied in each local environment with the engagement of diverse teams of people dedicated to improving the problem being addressed. Through a human-computing symbiosis, any measured outcome for any process can be improved.
As our team learned to apply these data science principles, we made many mistakes and overcame many challenges. But as we became more skilled over several years, we’ve seen the potential for our healthcare system to be transformed. The challenge ahead is ethically implementing a data and analytics infrastructure in healthcare that supports the ability of each local clinical environment to produce algorithms that improve outcomes over time.
Reframe Ethical Issues
The ethical issues about the use of artificial intelligence and machine learning in our world really have nothing to do with the technology—they’re about the humans who program the technology. The people who design programs may produce harm that is intentional or unintentional, but when the purposes of the algorithms that permeate our world are opaque and hidden, there is a void of accountability. The ethical solution is not only through gaining consent from individuals to use their data, but through the transparency and accountability of those who use and program the data. And, most important, the public must value that transparency and accountability without rigid judgment of failures.
We are at a critical moment in our world. Will we use our lower-brain mindset, designed for a world of scarcity and imminent physical threats, to use technology for selfish and competitive desires? Or will we use our higher cognitive capacity to create a world of abundance through collaborative uses of technology that result in a better world for all people? It’s up to us. Do we want to compete to prove we’re right, or do we want to learn to improve and do what is right? As when I closed my TEDx talk in 2012, I still believe the future of our world will be based on each person’s answer to one simple question: Do you care? I do.