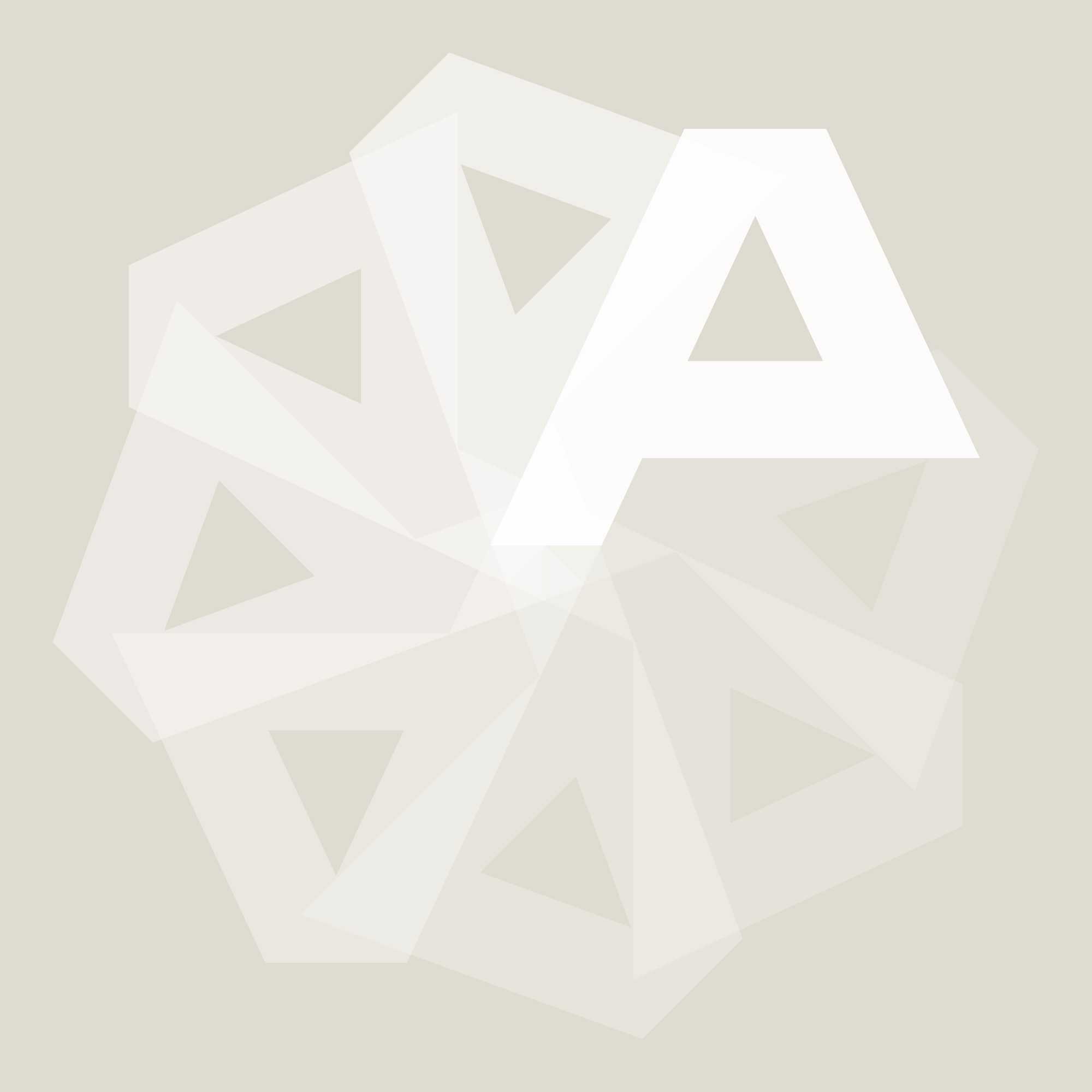
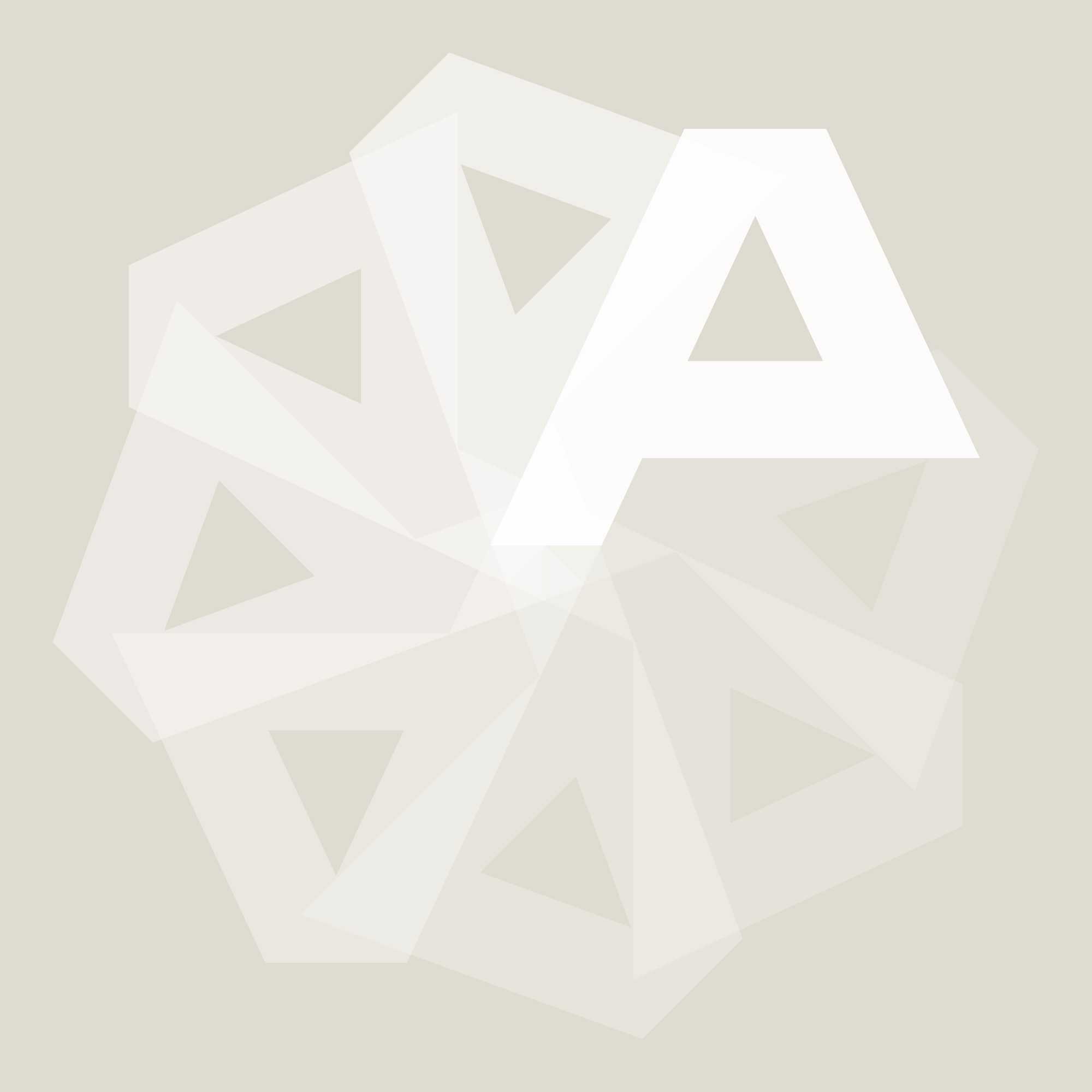
AI has a huge potential to revolutionize healthcare, but over-hyping AI can undermine the credibility of the promise.
Imagine a future where every single doctor makes decisions on diagnosis and treatment of a patient drawing from the real-time collective wisdom generated by all clinicians and the outcomes of billions of patients worldwide? In other words, ensuring that every patient gets the best care possible based on the most up to date science and evidence.
This is in essence the amazing promise of artificial intelligence (AI) and machine learning (ML) as highlighted in a recent review article in The New England Journal of Medicine.
Today, clinicians are faced with the insurmountable challenge of keeping up with the speed of medical knowledge and make sure they deliver the most up to date treatment and care to their patients. It is estimated that the doubling time of medical knowledge in 2020 will be just 73 days. Students who began medical school in the autumn of 2010 will experience approximately three doublings in knowledge by the time they complete the minimum length of training (7 years) needed to practice medicine.
AI represents a potential promise to overcome this challenge as AI and related technologies, such as machine learning (ML), provide the capability of augmenting decisions and predictions based on insights developed by ML-algorithms that are far superior to the insights generated by humans.
AI and ML offer the potential promise of helping clinicians and health systems deliver better clinical outcomes and reducing costs, thus becoming a panacea for struggling healthcare systems around the world under pressure from changing demographics, rising costs, growing patient expectations and the need to fund medical innovations for unmet needs.
It is not surprising that the healthcare industry is optimistic about AI, as demonstrated in a recent survey by Optum where health care executives from across the industry – in health plans, hospitals and health systems, life sciences and employer organizations – reveal an overwhelming belief that AI technology is the most reliable path toward equitable, accessible and affordable health care. In fact, the majority of those surveyed say that their organization is actively applying AI-strategies in various areas across their business.
Yet, the actual adoption of AI in medical practice is still debated, and many healthcare leaders remain skeptical about the speed by which AI and ML can be implemented on a larger scale to deliver value to patients and providers.
Hype and Over-promotion
While there is general consensus about the potential promise of AI and related technologies, such as ML and surgical robots, there is concern that these technologies are being over-sold and over-hyped, thus generating credibility issues and resistance to adoption among clinicians.
A major backlash for the AI community occurred in 2017, when an article in STAT revealed that IBM Watson was unable to deliver on its promise that the supercomputer could deliver a revolution in cancer care. STAT found that the system doesn’t create new knowledge and is artificially intelligent only in the most rudimentary sense of the term. The article also revealed that major cancer centers around the world had pulled out of collaborations with IBM Watson as they did not find that the supercomputer could deliver on its promise.
In fact, over-promise can create a trust problem. “The biggest barrier is overselling; you don’t want to oversell because then you lose trust,” says Aziz Nazha, who heads the Center for Clinical Artificial Intelligence that launched this spring at the Cleveland Clinic.
In many cases, AI and AI-related technologies are implemented by healthcare organizations and health systems without scientific evidence for improved clinical outcomes.
Surgical robots and robotic-assisted surgery are a case in point as these technologies often are implemented without prior documentation for their clinical benefits.
An article in JAMA earlier this year raised concerns that evidence to support the use of robotic-assisted surgery for the management of breast cancer and other cancers was limited and may even be associated with shorter long-term survival compared with other surgical approaches.
In a recent article in Dagens Medicin, the leading Danish daily healthcare journal, two leading surgeons criticized that some Danish regional health authorities have invested in robotic surgery without a clear plan for scientific documentation and clinical outcomes. They pointed to the application of robotic surgery in a simple surgical procedure such as hernia repair where the value of the robotic procedure is not clinically justified and in fact wasteful.
There is wide-spread concern that some hospitals make investments in robotic surgery to market their services and attract patients before they have made the evidence-based case for the value of the investment.
Roadmap for Hope
There is no question that AI, ML and robots are part of the future of healthcare, but what is the most meaningful roadmap for adoption?
- Advance scientific evidence and peer-review. Any major advance in medicine has to be based on scientific evidence and peer-review. The sharing of evidence through peer-reviewed publications is the foundation for the advancement of AI and AI-related technologies as it is with any other medical innovation. It is interesting that IBM Watson rejected calls for peer-review of the supercomputer’s cancer applications citing concerns about commercial IP. However, as Aziz Nazha from Cleveland Clinic points out, it is a challenge that it can be difficult to publish papers on AI in healthcare in scientific journals, since peer reviewers are often profoundly unfamiliar and uncomfortable with the subject. Leading medical journals need to overcome this challenge by identifying qualified reviewers to ensure that experience from applications of AI-models in clinical trials, patient care and public health can be shared and critically validated.
- Bridge the gap between digital technology and healthcare. While technology companies are at the forefront of investing in AI and related technologies, traditional healthcare organizations are still reluctant to embrace these technologies. There are a growing number of joint partnerships between technology companies and healthcare companies, such as for example the collaboration between Google’s Verily and Johnson & Johnson/Ethicon around robotic surgery, and such partnerships bode well for the future. However, many healthcare organizations and companies are still reluctant to engage more broadly in AI and ML. As an example, the Partnership on AI in the United States gathers a large number of technology companies, consulting firm and NGO’s, but the partnership does not have a single representative of the healthcare industry as a member – despite the fact the partnership is focused broadly on bringing together diverse, global voices to realize the promise of artificial intelligence across many topics, including economy, social, labor and health.
- Make high-quality data available. Ensuring that high-quality data is available is a major challenge for the wider adoption of AI and ML. Successful implementation of AI and ML require aggregation of a broad range of data from a variety of sources, but some data sources are not reliable, and the validation of data and linking of diverse data-sets can be an arduous process that requires highly skilled and trained experts with both data analytic and clinical experience. Earlier this year, the CEO of Novartis, Vas Narasimhan, explained the painful process of applying AI and ML in pharmaceutical R&D: “The first thing we’ve learned is the importance of having outstanding data to actually base your ML on. In our own shop, we’ve been working on a few big projects, and we’ve had to spend most of the time just cleaning the data sets before you can even run the algorithm. That’s taken us years just to clean the datasets. I think people underestimate how little clean data there is out there, and how hard it is to clean and link the data.”
- Motivate and incentivize clinicians. Many clinicians are on the fence when it comes to digital technology in medical practice. The biggest large-scale implementation of technology in medicine in the last decade, the electronic medical record, has been controversial and painful. While few clinicians oppose the principle benefits of the electronic medical record, it has been the source of workflow disruptions, burnout and has fueled excessive demands for data generation by clinicians that have created additional workload. If AI and ML are to be successful, health systems, policy makers and payers need to look at models for financially incentivizing and motivating clinicians to continuously deliver the high-quality data on their patients as the prerequisite for building high-value clinical databases and algorithms.
- Educate and communicate. The field of AI, ML and related technologies, such as robots, is highly complex as it involves understanding of computer science, algorithms, and their application in daily medical practice. This will require hands-on education in medical school as well as in post-graduate training, but it will also require that digital technology experts get educated to better understand medical science and clinical practice. Maybe, a new combined medical-digital education and job-profile needs to be designed and implemented to facilitate the needs of healthcare in the future. Simultaneously, the public at large should be educated about the benefits of AI in healthcare.
Reasons to Be Optimistic
There are reasons to be cautiously optimistic about AI in healthcare, but it will likely take a couple of decades before we will experience a broader application of this technology in daily medical practice. And let’s patiently support the advancement of AI and ML by fostering supportive scientific evidence, education, collaboration and communication.