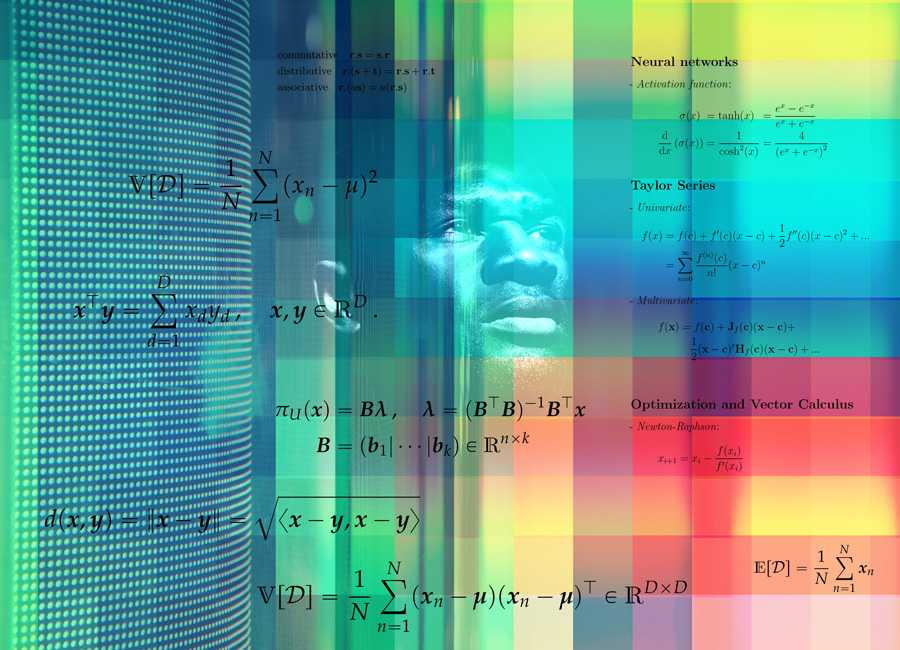
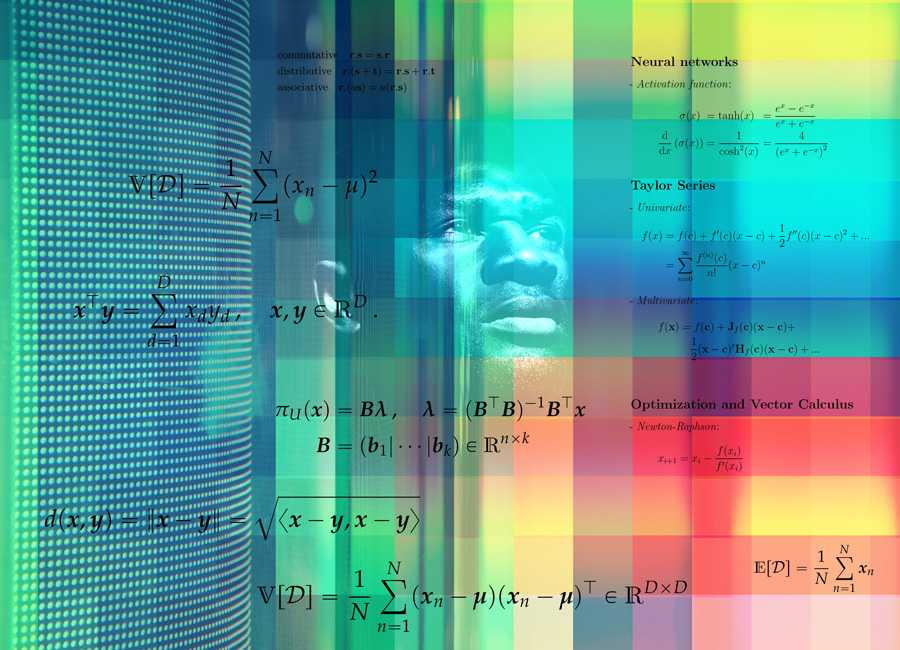
Companies and organizations continue to struggle with identifying and mitigating racial bias across the algorithms that autonomously decide who receives healthcare, predict future criminals, qualifies mortgages, who gets to college or who gets the job. In these and many cases, the algorithms are automating decision-making reasoning with their statistical correlation as they were trained. As these systems learn from experience, the outcomes of their decisions become a self-reinforcing loop, and the underpinning data perpetuates discrimination and societal biases thus becoming the data that feeds racism.
Data mining is no longer a goldrush but a constant sprint to turn data into knowledge and knowledge into insights. But looking for answers without enforcing fairness in data due process to mitigate racial and prejudicial biases carries an inherited business risk that acts as technological harm, embeds systemic racism and open business to reputational risk.
We see racial bias models on systems gone wrong at large scale, often unnoticed like on interfaces that are familiar to us like automatic photo cropping that is more favorable to white faces or physical products like a pulse oximeters that are biased by design and are less accurate for Black people.
The sources of racial bias in data, in addition to human decisions, are sensors in our phones, cameras, other smart devices and infrastructure, language generation across broadcast, social and traditional media, application forms and voice enable devices, and transactional data generated by commerce and financial institutions. Collecting unfiltered data introduces cultural artifacts, stereotypes and prejudice. Executive management should be aware of the risks and implications associated with unfair data practices.
The data drives analytics, machine learning models and artificial intelligence put into production and have significant ethical and moral dimensions that should be part of project risk assessments and algorithmic audits for data science and management teams—such as human rights and the imperative not to not erase underrepresent segments of the population. Ethical dimensions of impartiality and fairness requires developing the human sensitivity needed to understand how racial and prejudice affect data samples and how it impacts the lives of all people and their future.
The knowledge and insights are generated by the training data that influences the intuition of trained models, the level of accuracy of mathematical discrimination must be corrected and failsafe enabled as well. To do so, organizations should deeply consider the following steps:
- Transend the belief that our technology is neutral. People and data are biased; the machine learning algorithms using that data will inevitably be biased. There is no such thing as an objective state. Our data reflect our human reality and beliefs.
- Proactively identifying the unintended consequences of algorithms (what-if scenarios) should be a default exercise of data science team and crisis plan.
- Foment diversity of thought and thinking in your data intake and science process. Interdisciplinary team are best poised understand how to manage the sensitivities of handling “sensitive attributes” such as race, gender and socio-economic dimensions.
- Scrutinize shortcuts on development time, often introduced by implementing open source algorithms. Establish data pre-processing and fairer data methods.
- Adapt and redesign outdated business processes that need to accommodate fairness.
- Ask the important questions—what is the worst thing the algorithm could do? Are you ready to be in the national headlines? Have you thought of the impact of regulation?
These steps are just a start to accelerate what’s right with data ethics and to unleash the positive economic performance and experiences for consumers and citizens alike.
Great knowledge and insights come with great responsibility and as business and organizations select how to use data towards their business activities, none can claim data neutrality. Identifying, avoiding and mitigating racial data bias should be criteria for stakeholders and indications of long-term value. There are clear business and cost-effective strategies to mitigating racial and prejudice bias, but above all, these offensive applications affect people’s lives and should be open to scrutiny and accountability.
We have control over what and how data affects the algorithms we build and that can make the difference on our bottom-line but above all, we can be fairer to all people.